AL & ML
Artificial Intelligence & Machine Learning
CloudSight Technologies offers a wide range of healthcare solutions that have deep AI technological capabilities. Case in point, the firm has a basing Prediction, Diaganosis recommendation system on a Patients care journey through the AI Care Pathway System, which is now in its First year of advancement. As a result, the recommendations are now next-action-prediction based rather than simple “Diagnosis” recommendations that most early recommender systems provide.
CloudSight Technologies offers a wide range of healthcare solutions that have deep AI technological capabilities. Case in point, the firm has a basing Prediction, Diaganosis recommendation system on a Patients care journey through the AI Care Pathway System, which is now in its First year of advancement. As a result, the recommendations are now next-action-prediction based rather than simple “Diagnosis” recommendations that most early recommender systems provide.
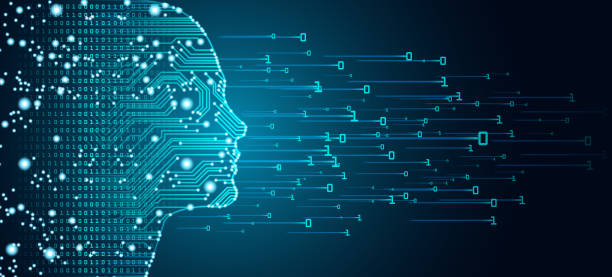
CloudSight Technologies also Offers AI based Pre-Authorization and Claims management systems. CloudSight Technologies also offers an AI-based differential diagnosis at the forefront of patient-doctor conversations. The objective is not only to reduce physician workloads but also to restore the human touch between patients and doctors.
The company recently published an article — the probabilistic model for a differential diagnosis: development and validation of a probabilistic model to address the lack of large-scale clinical datasets.
Doctors in the country are overburdened. There is an acute shortage of healthcare force which is projected to worsen in the coming years. The company is striving to develop a model that will
- Make good quality healthcare available to every person in the society even those residing in the remote corners of the country
- Improve the efficiency of the doctors
- Ensure and accentuate the quality of healthcare delivered
The consultation carried out by a doctor to assess our health problem has two main parts: information collection to reach a diagnosis followed by management of the health issue. Our team figured out that there is a lot of scope of technology-based interventions in the first part of information collection. This information gathering can be further subdivided into history taking (this is the part where the doctor asks questions about our symptoms and complaints), physical examination and investigations. In a large percentage of patients, a medical history alone is sufficient to determine a list of differential diagnosis without the need for investigative evaluation. Thus, the company decided to focus its attention to this part of the problem.
CloudSight Technologies tried different approaches, over last quarter, and reached the conclusion that the art of diagnosis based on history is not a one-to-one connection but a mix of different possibilities. This gave birth to the idea of using a Bayesian approach to create the probabilistic model for diagnosis.
During history taking, symptoms are medically relevant information obtained from the patient. For the model, 1mg defined values as the objectification of the patient response, for symptoms and their characteristics, elicited by the patient. The possibilities of a response value are discrete and restricted by symptoms.
Evidence (e) is defined as a set of symptom-value pairs based on the current complaints of the patient. The universe of all possible symptom-value pairs is E, and every patient presents with a subset of E. The addition of every new symptom results in the probability of disease being modified; that is, the prior probabilities are updated with the addition of new evidence. Thus, by aggregating all the probabilities, the model comes up with a list of differential diagnoses for the current complaints of the patient.
In the study, CloudSight Technologies used clinical charts of patients (created by Internal Medical specialists) and gave them to six doctors and the model. Diagnosis and differential diagnoses for the patient’s condition given by the doctors and model were compared over predefined metrics. Results demonstrated that the model performed at par with the doctors.
Similar studies have been conducted previously too by many researchers, but this was the first time that such results have been seen for a model. Our study demonstrated the power of probabilistic modelling for medical diagnosis when the local patient and disease profile was kept in mind rather than utilising an off-the-shelf approach.
In addition, we believe extensive involvement of practicing clinicians during the development phase is essential for the creation of a solution with demonstrable accuracy and clinical relevance.
CloudSight Technologies also Offers AI based Pre-Authorization and Claims management systems. CloudSight Technologies also offers an AI-based differential diagnosis at the forefront of patient-doctor conversations. The objective is not only to reduce physician workloads but also to restore the human touch between patients and doctors.
The company recently published an article — the probabilistic model for a differential diagnosis: development and validation of a probabilistic model to address the lack of large-scale clinical datasets.
Doctors in the country are overburdened. There is an acute shortage of healthcare force which is projected to worsen in the coming years. The company is striving to develop a model that will
- Make good quality healthcare available to every person in the society even those residing in the remote corners of the country
- Improve the efficiency of the doctors
- Ensure and accentuate the quality of healthcare delivered
The consultation carried out by a doctor to assess our health problem has two main parts: information collection to reach a diagnosis followed by management of the health issue. Our team figured out that there is a lot of scope of technology-based interventions in the first part of information collection. This information gathering can be further subdivided into history taking (this is the part where the doctor asks questions about our symptoms and complaints), physical examination and investigations. In a large percentage of patients, a medical history alone is sufficient to determine a list of differential diagnosis without the need for investigative evaluation. Thus, the company decided to focus its attention to this part of the problem.
CloudSight Technologies tried different approaches, over last quarter, and reached the conclusion that the art of diagnosis based on history is not a one-to-one connection but a mix of different possibilities. This gave birth to the idea of using a Bayesian approach to create the probabilistic model for diagnosis.
During history taking, symptoms are medically relevant information obtained from the patient. For the model, 1mg defined values as the objectification of the patient response, for symptoms and their characteristics, elicited by the patient. The possibilities of a response value are discrete and restricted by symptoms.
Evidence (e) is defined as a set of symptom-value pairs based on the current complaints of the patient. The universe of all possible symptom-value pairs is E, and every patient presents with a subset of E. The addition of every new symptom results in the probability of disease being modified; that is, the prior probabilities are updated with the addition of new evidence. Thus, by aggregating all the probabilities, the model comes up with a list of differential diagnoses for the current complaints of the patient.
In the study, CloudSight Technologies used clinical charts of patients (created by Internal Medical specialists) and gave them to six doctors and the model. Diagnosis and differential diagnoses for the patient’s condition given by the doctors and model were compared over predefined metrics. Results demonstrated that the model performed at par with the doctors.
Similar studies have been conducted previously too by many researchers, but this was the first time that such results have been seen for a model. Our study demonstrated the power of probabilistic modelling for medical diagnosis when the local patient and disease profile was kept in mind rather than utilising an off-the-shelf approach.
In addition, we believe extensive involvement of practicing clinicians during the development phase is essential for the creation of a solution with demonstrable accuracy and clinical relevance.